Jennison Perspectives - Artificial Intelligence: The Great Wave
October 30, 2023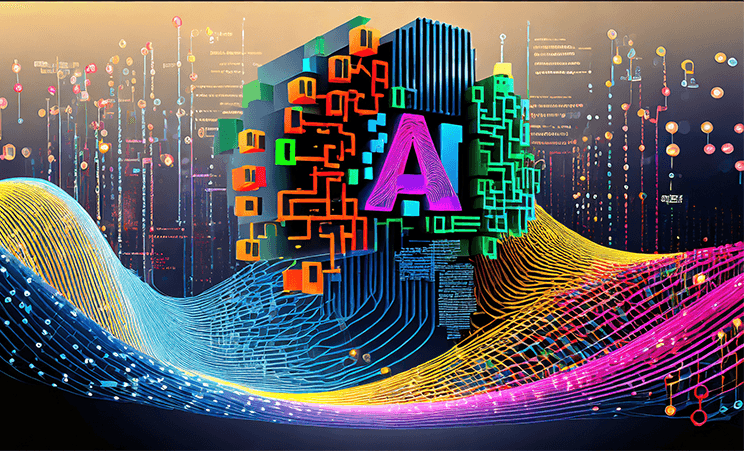
According to Harbor’s subadvisor, Jennison Associates, the wave of artificial intelligence (AI) advancements and applications commands attention. In the piece below, Jennison discusses the following observations:
- We are in the opening stages of a generative AI-driven investment cycle that we believe will sweep through the technology sector and, ultimately, the economy.
- The scope of this transformation can be extraordinary for companies, which will need to effectively incorporate generative AI into their business models to compete.
- While this opportunity is still in its beginning stages, investors can use Jennison’s framework to understand the investment implications of generative AI and its development over time.
The Hype is Real
We believe recent advances in AI herald a technological transformation, one that will be as significant and long lasting as the advent of the internet and mobile computing. Within the next several years, we expect to see generative AI use cases and applications spread from technology providers and developers to a wide range of industries. Companies can use these tools to harness data in increasingly sophisticated ways, which could help increase competitive positioning, improve time to market, and streamline customer service. For well-positioned, well-managed, and adaptable companies, in our view, the scope and depth of the opportunity is extraordinary.
It is understandable that some investors dismiss this as technology hype — especially since generative AI does not actually think as much as it uses data, statistics, and algorithms to make a prediction. In our view, however, the AI revolution is real and is just beginning. As we explore below, demand for the infrastructure components necessary to support generative AI models has already soared, and we expect the full impact of generative AI will be like a wave sweeping through the economy (Exhibit 1). To capitalize on this opportunity, we think investors need a deep understanding of the AI ecosystem, where it is today, and the full implications of its future development.
Some investors dismiss AI as technology hype – but we believe the AI revolution is real and is just beginning.
Exhibit 1: The AI Wave is Sweeping through the Economy
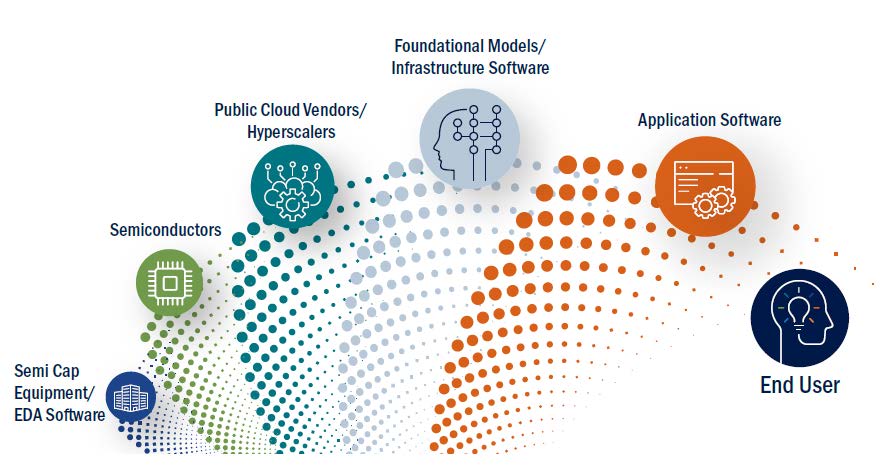
For illustrative purposes only. Source: Jennison Associates.
What is Generative AI?
Generative Artificial Intelligence (generative AI) is a subset of AI technology. Generative AI can create new and unique content, including text, images, video, music, and computer code. This content is often indistinguishable from those created by humans. By contrast, traditional or predictive AI systems are predominantly deterministic and rule based. They are excellent at following explicit guidelines to perform tasks, but traditional AI lacks the capacity for creative or “novel” output. Both traditional and generative AI leverage complex algorithms and are trained on extensive data sets.
Generative AI achieved a major breakthrough in November 2022, when OpenAI publicly released a large language model (LLM)—ChatGPT-3.5. The LLM was “trained” on an expansive data set that included sources such as the Bible, encyclopedias, blogs, and newspaper articles. By analyzing billions of sentences and paragraphs, the model learned the underlying patterns of grammar, context, and word usage. The trained model could then use “inference”— i.e., its knowledge of language — to answer prompts with original content, whether it be a summary, an essay, or even a tagline for an ad campaign. ChatGPT offered the power of generative AI to everyday users, which is reflected in its rapid adoption rate (Exhibit 2).
Generative AI can be applied to virtually any data set — words, numbers, images — and it can take on a range of tasks that require flexibility and judgment. Moreover, the models learn from additional data and improve with usage, so we are just starting to grasp generative AI’s true potential.
Exhibit 2: AI Technology Has Been Adopted at Historic Speed
Months it took selected apps to reach 100 million monthly users
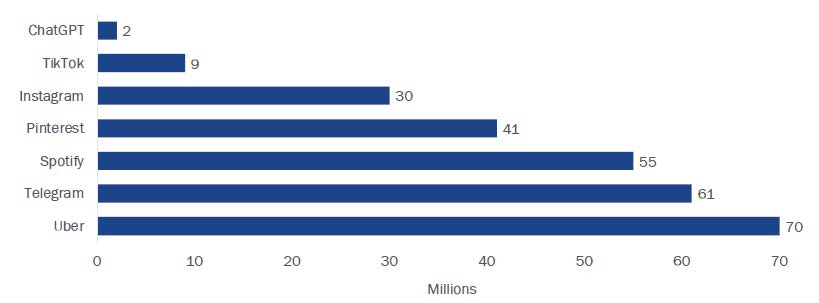
Source: Statista, 2023
Today’s AI Investment Opportunities
Semiconductors, Semi Cap Equipment, and EDA Software
We believe one of the most tangible ways to invest in generative AI today is through the semiconductors and infrastructure needed to support AI model training and running scaled AI-enabled applications. Graphic processing units (GPUs) are the core technology, combining cutting-edge semiconductors and customized software to process multiple computations simultaneously. Demand for these components rose sharply in 2023. Upward revenue revisions for Nvidia in 2023 — the leading GPU producer — were among the largest we have ever witnessed. Bill Dally, Nvidia’s chief scientist, believes “training requirements [for the most advanced AI systems] will continue to double every six to 12 months.”i
Surging demand for semiconductors reflects a sense of urgency among companies that they must incorporate generative AI into their business models.
In addition to GPU manufacturers, other semiconductor companies — such as analog chip makers and memory companies — will likely benefit, in our view. Analog semiconductors read real-world signals — e.g., temperature, light, heat, sound — and translate them into the data used in training models. Memory chips are needed to store these vast amounts of data. The growing usage of semiconductors across these roles, in turn, has driven demand for the semi cap equipment companies that create the sophisticated hardware needed to manufacture chips. We are seeing innovative use of materials and packaging technologies to deal with the thermal demands of AI compute as well.
Electronic design automation (EDA) software companies have also become increasingly important to the semiconductor industry. EDA companies create the software that allow semiconductor engineers to design chips that are smaller, more powerful, and require great power efficiency. As more and more functionality is packed into smaller and more complex chips, semiconductor engineers are turning to EDA software to optimize chip layout, and they are using more simulation tools from the EDA vendors to test how these designs will hold up under various conditions. In short, EDA companies have become critical partners for semiconductor manufacturers. The customer base for EDA companies is expanding to include large technology and consumer electronics companies, which need EDA and generative AI expertise as they design their own chips specifically for customized tasks.
EDA companies are using generative AI in their own products as well. Among other improvements, EDA companies use historical design data to train models that can create new and novel chip designs with more efficient layouts. This continued innovation has allowed them to increase their share of semiconductor company research and development budgets. Their software is becoming irreplaceable in the design process, and human design talent is more difficult to scale up.
Surging demand for semiconductors reflects a sense of urgency among companies, from hyperscalers to small enterprises, that they must incorporate generative AI into their business models or potentially lose market share. This urgency is driving an investment cycle (Exhibit 3).
Exhibit 3: The Market for Generative AI is Just Beginning to Grow
Forecast for generative AI market ($/billions)a
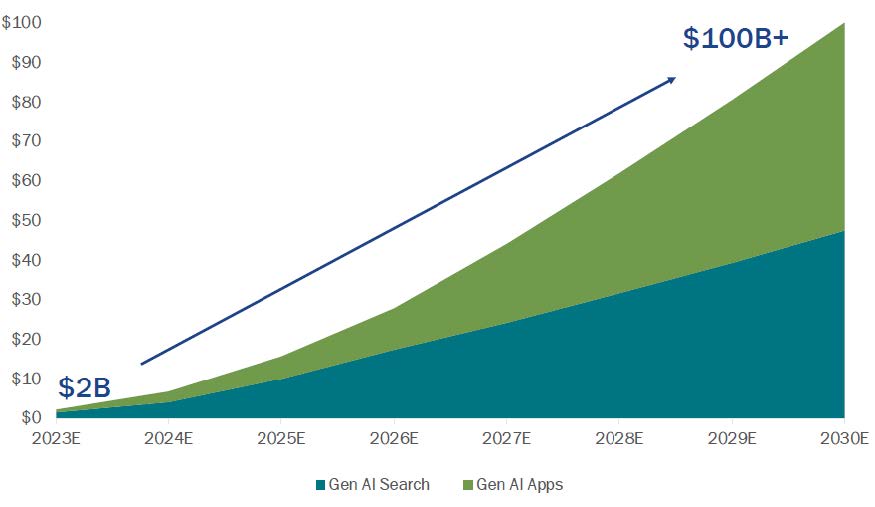
a Forecasts (E) may not be achieved and are not a guarantee or reliable indicator of future results. Although Jennison believes that the expectations reflected in such forward-looking statements are based on reasonable assumptions, actual results may differ materially from those projected. Source: Piper FactSet, Company Filings, Piper Sandler Estimates, Piper Sandler Research
Hyperscalers/Public Cloud Vendors
The AI wave is also reaching the hyperscaler cloud vendors, who provide cloud computing infrastructure (e.g., Microsoft Azure, Google Cloud, and Amazon Web Services). Hyperscaler cloud vendors effectively build massive datacenters filled with servers and rent out that capacity to customers. These vendors are some of the largest buyers of semiconductors and we expect them to continue to heavily invest in building out GPU capacity. We believe demand for this capacity should rise as businesses seek computing power to train their models and run their AI applications in production. The economies of scale created by these vendors are especially valuable to smaller companies, who may not have the resources to purchase these compute resources directly. As such, we see hyperscaler cloud vendors as enablers of the technology infrastructure that will bring generative AI to a broad market over time.
Foundational Models and Infrastructure Software
Foundational models (or base models) are large machine learning models that are trained on extensive data sets with parameter counts that are frequently in the tens of billions. They are “foundational” because they can be adapted to a wide variety of tasks/functions such as educating, image creation, and coding. The models can vary in size, depending on the task or the device or server on which it will be run. Foundational models often serve as a starting point for more specialized models that can be used to perform more focused or industry-specific tasks. These more specialized models are often created using a process called “fine tuning,” and they can often perform faster and save computational resources. Furthermore, foundational models can be augmented with propriety data using a variety of techniques — such as “retrieval augmented generation” (RAG) — to enhance their capabilities beyond the data they were originally trained on. These techniques can make foundational models even more useful in everyday operations for industries and enterprises with unique requirements.
Foundational models come in many different flavors, including for text, images, video, and audio. Foundational models can also be multi-modal—they can interpret and generate multiple types of data. For example, a multi-modal model could record a conference call, transcribe the audio into text, graph each participant’s time speaking, list the topics of discussion, and calculate their duration on the call. Another example is a robotic arm with a video camera mounted on top. The robot is given a voice command, for example: “pick up the red wood block.” The multi-modal model could transcribe the audio into text; convert the text into computer code in real time; and use the code to direct the robotic arm to identify the block through the video camera and pick it up.
Infrastructure software includes the tools and data management systems that software developers use to build their own generative AI applications. These companies provide the “technological plumbing” that enables enterprises to run applications and services, allowing them to tap into generative AI functions and get insights on their data. The development of any new application must incorporate infrastructure software. Generative AI applications also have new and specific requirements compared to traditional applications, and we believe providers of those services will be significant beneficiaries of the coming wave.
Application Software
Application software is used by an end user to perform a specific set of tasks and/or to solve a particular problem. It is used by both consumer and business users. Some examples of application software are Microsoft Excel and Workday. Common examples of consumer application software include Google Maps and Spotify.
ndividuals, businesses, and enterprises interact with generative AI through their use of application software. The most successful consumer-facing generative application in the market, ChatGPT, offers users access to OpenAI’s LLM. We believe that while there is an enormous opportunity for many other consumer-facing applications to utilize generative AI, the opportunity in enterprise applications is even larger. Businesses will gain much more from specialized use cases, which we expect will drive massive productivity gains. Application software vendors will be able to share in those economic benefits, in our view.
Business applications for generative AI are already in use — and many more are being developed — throughout the economy. Software companies are already reporting higher productivity as software engineers use generative AI to draft code. Marketing software could generate drafts of content, pulling from a proprietary database of content/creative collateral or even company guidelines for additional context. It could localize the material for a global campaign, taking into consideration cultural differences between regions and countries. Customer service departments could handle inbound calls, emails, and voice inquiries through a model that draws on the firm’s internal guidelines and knowledge. Product designers could simply describe what they want to create, and generative AI applications could generate options for the designer to consider and further refine. Large banks or insurance companies could use generative AI to improve risk underwriting through the analysis of large sets of data and consumption patterns, focusing on consumer risk and fraud. Entertainment companies can use generative AI to compose music, write first drafts of scripts, and create moving images with dialogue. In medicine, it can scan test results, diagnose disease, and recommend treatments. Generative AI can also detect inefficiencies, accelerate drug discovery (and generate new molecules), and improve clinical-trial planning and execution.
For individual users, we believe a transformative moment will occur when generative AI applications can interact with user tools.
For individual users, we believe a transformative moment will occur when generative AI applications can interact with user tools — such as browser, calendar, email, office and entertainment apps. Apps can assist the user to complete personal or business-related tasks: to draft a memo, or organize a spreadsheet, or lay out a presentation. Or, the model could plan a family vacation, order ingredients for a gourmet meal, or recommend a gift for a 12-year-old nephew. Ultimately, this will make the end user more productive, which in turn raises demand for generative AI and the infrastructure that makes it possible — completing a virtuous circle and starting the wave all over again.
Evidence has already emerged that investments in generative AI are starting to pay off. Even though many of these efforts are still in a test phase, companies have reported that generative AI has improved their businesses, such as in product development or customer service analysis. We expect those who are providing these enhanced capabilities should be able to price for the value created.
Data Purity
Companies with access to large, high-quality sets of proprietary data can also benefit from generative AI. Models require extensive sets of data for training, but they also require data to yield insights that can improve businesses. As a result, non-technology companies with strong data architecture and deep pools of data — healthcare is especially interesting, in our view — are positioned to be lead beneficiaries of generative AI.
Controversies and Challenges
Major technological change does not occur without challenges, delays, and setbacks. Generative AI has drawn a number of critics, who see the technology as potentially destabilizing, dependent on global trade, prone to mistakes, and a ravenous consumer of natural resources.
Generative AI has driven speculation that it will replace millions of jobs across the economy, sparking upheaval and unrest. In our view, it is more likely to take over repetitive, mundane tasks and free up workers to focus on more important challenges. This could lead to significant advances in productivity and automation and help address looming labor shortages. A law firm found that its generative AI program could perform lower-level tasks but needed to be monitored. The law firm considers the technology “a boring productivity gain. We know it has issues, we know it makes errors, we know it will be out of date. But that’s OK, because we’re trying to save an hour or two a week across 3,500 people who have access today.”ii
We are concerned about de-globalization. Rising geopolitical tensions threaten the supply chains and access to markets that have so far been crucial to the expansion of AI.
We are more concerned about de-globalization and rising geopolitical tensions — especially around semiconductors — which threaten the supply chains and access to markets that have been crucial to the expansion of AI. Another potential challenge is government regulation, which, given the reach and broad implications of generative AI, is unavoidable. However, the complexity of generative AI, the fact that it is still in early stages, and the potential of unintended consequences, raises the risk that regulation will be ineffective or impede development. Generative AI also requires considerable energy resources, and we believe the industry’s growth must be efficient or its carbon footprint will draw the critical scrutiny of ESG-inspired investors.
Open- or Closed-Source Models?
Generative AI is being developed through both open-source models and software versus proprietary closed-source models. Some large tech companies have invested heavily in proprietary, closed generative AI models that they hope will become major sources of revenue. These companies do not disclose model weights, training data, or techniques. On the other hand, open-source models are becoming popular because they are widely available and can be modified with relative ease. Open source can also move faster because it attracts a large community of developers, who vet the software and often improve it (or fix bugs if necessary). We expect a mix of open- and closed-source models will emerge over time depending on the use case, however the establishment of standards will have major implications for company business models in the generative AI ecosystem.
A Historic Transformation – A Historic Opportunity
We expect generative AI will meet and ultimately surpass these challenges. In fact, the generative AI wave is only beginning to sweep through the economy and society, and it can be difficult to fully appreciate its scope and potential. Investors, in our view, should consider the last time technology attracted such interest — the 1990s. At that time, the tech boom brought strong support for new companies. It took time, however, for the true winners with sustainable business models to emerge, and the markets suffered a reversal before the promise of the tech revolution was delivered. We believe the environment is similar today.
Long-term structural change in the economy does not happen every day — our goal is to be there when it does.
Long-term structural change in the economy does not happen every day — our goal is to be there when it does. We are watching the AI transformation closely. It is difficult, at this stage, to identify the companies that will develop the next generation of generative AI applications for end users. We are more confident that, while there will be many failures, the companies that successfully use generative AI will have extraordinary advantages. We have been applying our approach to growth investing for more than five decades, and we believe that our commitment to fundamentals, our deep resources, and our experience investing through multiple cycles of economic transformation leave us well positioned to select the next generation of innovators.
Important Information
i Richard Waters and Qianer Liu, “Why China remains hungry for AI chips despite US restrictions,” Financial Times, August 21, 2023.
ii Sarah O’Connor, Christopher Grimes and Cristina Criddle, “AI shakes up way we work in three key industries,” Financial Times, June 18, 2023.
The views expressed herein are those of Jennison Associates at the time the comments were made. These views are subject to change at any time based upon market or other conditions, and the author/s disclaims any responsibility to update such views. These views may not be relied upon as investment advice and, because investment decisions are based on many factors, may not be relied upon as an indication of trading intent. The discussion herein is general in nature and is provided for informational purposes only. There is no guarantee as to its accuracy or completeness.
Investing entails risks and there can be no assurance that any investment will achieve profits or avoid incurring losses. Stock markets are volatile and equity values can decline significantly in response to adverse issuer, political, regulatory, market and economic conditions.
References to specific company are for illustration only and should not be construed as an offer or solicitation from Harbor Capital to buy or sell any securities.
Hyperscalers are large cloud service providers, that can provide services such as computing and storage at enterprise scale.
3195857